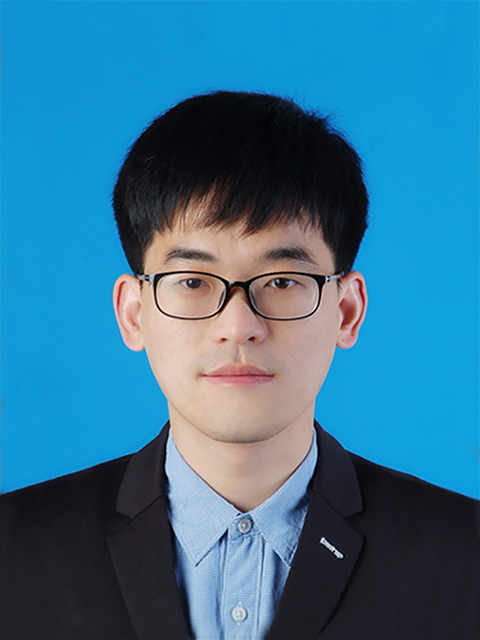
Shichao Jin, Associate Professor at NAU and Principal Investigator of JinLab, Master's Supervisor
Email:jschaon@njau.edu.cn
Tel:025-84396112
Address:Room 306, No.3 Experimental Building, Nanjing Agriculture University
Education and Employment
2020-Present, Associate Professor in PPRC, NAU(High-level Talent Program)
2016 -2020, PhD in Ecology,University of Chinese Academy of Sciences
2012-2016, Bachelor in Forestry, Huazhong Agriculture University
Research interests
1) Intelligent extraction of plant targets and traits from lidar data using deep learning
2) Multi-source remote sensing data fusion and model coupling in the field of plant phenomics
3) High-yield plant phenotype screening and management optimization using remote sensing and crop models
Honors and Prizes
2019, Outstanding Youth Award. 2019 National Graduate Conference on Laser Remote Sensing and Probing, Peking University
2018,1stPrize Winner (1/800) of the Second International Student Academic Forum, Institute of Botany, Chinese Academy of Sciences
2016,National Top Ten Graduates of Forestry (undergraduate group,< 1/1000), Chinese Society of Forestry & National Forestry Administration
2016,The Liang Xi Outstanding Student Award (< 1/1000), National Forestry Administration
2012-2015, National Scholarship (Three consecutive years), Ministry of Education of the People's Republic of China.
2014,1stprize (< 1/1000) of college students' biological experiment skill competition in Hubei province, Department of Education, Hubei Province.
Selected Invited talks
Jin S., Su Y., Song S., Wu F., Pang S., Guo Q. Field high-throughput maize phenotyping using Lidar and deep learning,2019 National Graduate Conference on Laser Remote Sensing and Probing, China. 2019 (Oral, Invited)
Jin S., Su Y., Guo Q. A Deep Learning Pipeline for High-Throughput Maize Phenotyping from Lidar Data,International Student Research Forum, Denmark. 2019 (Oral, Selected)
Jin S.,Su Y., Guo Q.Vegetation mapping using crowdsourcing methods.Chinese Research Academy of Environmental Sciences. Beijing, China. 2019. (Oral, Invited)
Jin S., Su Y., Hu T., Guo Q. A deep learning pipeline for high throughput crop phenotyping. AGU. Washington, D.C., USA. 2018 Fall. (Poster)
Jin S., Su Y., Wu F., Guo Q. A deep learning pipeline for high throughput crop phenotyping. The second Asia-Pacific Plant Phenotyping Conference. Nanjing, CHINA. 2018. (Oral)
Selected Projects
High-level talent introduction project of Nanjing Agricultural University, 2020-2024,PI
The Strategic Priority Research Program of the Chinese Academy of Sciences, “Research and development of nondestructive phenotypic system”, 2013-2018,Participate
National Science Foundation of China, “Research on the extraction of three-dimensional phenotypes of crop based on lidar and deep learning technologies”, 2018.1-2018.12, Participate
National Science Foundation of China, “Analysis of the spatial pattern and driving force of the three-dimensional architecture of trees based on lidar technology: a case of Quercus mongolica”, 2019-2022, Participate
TheStrategic Priority Research Program of the Chinese Academy of Sciences,“Mapping the vegetation map of China at the scale of 1:500,000”,2018-2023,Participate
Academic Achievements
Dr. Jin focuses on the study of high-throughput plant phenotyping and multi-spatiotemporal applications using lidar remote sensing (Remote Sensing 2018, 10: 1183-1203) and deep learning technologies (Science China Earth Sciences, 2020, 1-18). (1)Dr. Jin firstly proposed a field individual plant segmentation algorithm based on deep learning technology(Frontiers in Plant Science 2018, 9: 866-875. first author). On this basis, he further broke through the challenge of fine-scale maize organ segmentation using deep learning algorithm (IEEE Transactions on Geoscience and Remote Sensing 2019, 58: 2644-2658. cover paper); (2) Innovatively proposed an organ skeleton extraction method based on computer geometric graphics (IEEE Transactions on Geoscience and Remote Sensing 2018, 57: 1336-1346), and took the lead in realizing high-throughput trait extraction at individual plant, stem, and leaf levels; (3) Systemically evaluated the effectiveness of maize aboveground biomass estimation (Plant Method, 2020, 69-87) with lidar-derived phenotypes at multi levels (from population to organ), and comprehensively proposed the best model and traits selected for biomass estimation. More studies about deep learning in plant phenotyping are introduced in our review paper (Science China Earth Sciences, 2020, 1-18), which was reported by many famous medias, such as ScienceNet and EurekAlert.
The above-mentioned interdisciplinary researches with deep learning technology were commented as an effective method of identifying crop organs by the top journal in remote sensing (Sun et al., 2020. ISPRS Journal of Photogrammetry and Remote Sensing, 195-207.“Using such a method, it could be feasible to delineate cotton boll locations on each branch if a cotton plant branch pattern is obtained (Jin et al., 2018)”), and was also recognized as an effective method for extracting phenotypic traits by the top journal in breeding (Ramstein et al., 2019. Theoretical and applied genetics, 132:559-567. “While neural networks, especially CNNs, are promising for automatically predicting component traits from image data on a single instance. One example comes from Jin et al. (2018) who used region-based CNNs to detect maize stems from field images”).
Patents
Jin S., Dian Y., Wang P., Teng M., Meng F., Peng L. 2018. A method and system for canopy cover calculation based on digital images. No: CN201610049382.6 (In Chinese)
Selected Publications (During 2018-2020)
(Please find more on https://www.researchgate.net/profile/Shichao_Jin/publications)
Jin, S.,Su, Y., Gao, S., Wu, F., Ma, Q., Xu, K., Ma, Q., Hu, T., Liu, J., Pang, S., Guan, H., Zhang, J., Guo, Q.2019.Separating the structural components of maize for field phenotyping using terrestrial lidar data and deep convolutional neural networks.IEEE Transactions on Geoscience and Remote Sensing.58(4): 2644-2658. (Cover Paper, JCRQ1)
Jin, S.,Su, Y., Wu, F., Pang, S., Gao, S., Hu, T., Liu, J., Guo, Q*. 2018. Stem-leaf segmentation and phenotypic trait extraction of individual maize using terrestrial LiDAR data.IEEE Transactions on Geoscience and Remote Sensing.57(3): 1336-1346. (JCRQ1)
Jin, S.#, Su, Y.#, Gao, S., Hu, T., Liu, J., Guo, Q*. 2018. The Transferability of Random Forest in Canopy Height Estimation from Multi-Source Remote Sensing Data.Remote Sensing, 10(8): 1183-1203. (Co-first author,JCRQ1)
Jin, S.,Su, Y.*, Gao, S., Wu, F., Hu, T., Liu, J., Li, W., Wang, D., Chen, S., Jiang, Y., Pang, S., Guo, Q*. 2018. Deep Learning: Individual maize segmentation from terrestrial Lidar data using Faster R-CNN and regional growth algorithms.Frontiers in Plant Science, 9: 866-875.(JCRQ1)
Jin, S., Su, Y., Song, S., Xu, K., Hu, T., Yang, Q., Wu, F., Xu, G., Ma, Q., Guan, H., Pang, S., Li, Y., Guo, Q. 2019.Non-destructive estimation of field maize biomass using terrestrial lidar: An evaluation from plot level to individual leaf level.Plant methods16(1): 69-87.(JCRQ1)
Guo, Q#., Jin, S#.Li, M., Yang, Q., Xu, K., Ju, Y., Zhang, J., Xuan, J., Liu, J., Su, Y., Xu, Q., Liu, Y. 2020. Deep learning application in ecological resources: theory, tool, application and challenges.Science China Earth Sciences,DOI:10.1007/s11430-019-9584-9(Co-first author,JCRQ2).
Jin, S.,Su, Y., Zhao, X., Guo, Q. 2019.A Point-Based Fully Convolutional Neural Network for Airborne Lidar Ground Point Filtering in Forested Environments.IEEE Journal of Selected Topics in Applied Earth Observations and Remote Sensing. 13: 3958-3974. (JCRQ1)
Jin, S.,Hu, T., Su, Y., Ma, Q., Guan, H., Yang, M., Guo, Q. 2020. “LiVegetation” system: A new investigation tool for vegetation mapping in the era of citizen science. Science China-Life Science (Accepted).